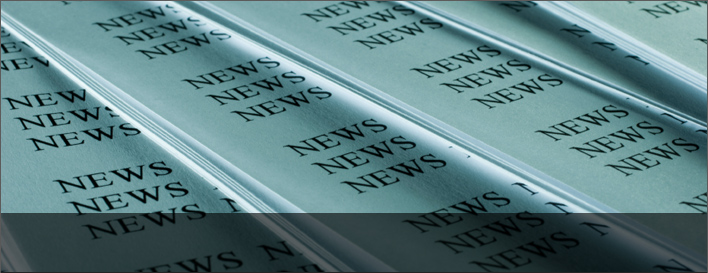
Machine Learning Algorithms Tested to Reconstruct the Historical Monthly Precipitation over Oklahoma
EPSCoR Update - October 2023
Machine Learning Algorithms Tested to Reconstruct the Historical Monthly Precipitation over Oklahoma
Oklahoma (OK) is a highly dynamic and variable region in the southern Great Plains that is vulnerable to drought. Major drought events in the early to mid-1900s and most recently in 2011 had caused economic losses affecting many Oklahomans. Consequently, long-term prediction of future precipitation is an important factor for drought management.
General Circulation Models (GCMs), or climate models, are important tools to predict future changes in temperature and precipitation in long range. GCMs coupled with dynamical land surface, oceanic, and atmospheric components, allow a comprehensive understanding of the Earth System.
“The GCMs’ precipitation projections, however, are subject to uncertainty arising from various sources such as model structures, parameterization schemes, simulation boundary conditions, etc. As a result, significant biases are associated with each individual GCM,” Dr. Tiantian Yang said.
“To obtain more accurate future climate projections, various multi-model ensemble techniques can be used to reduce uncertainties brought by each individual GCM. Through perturbations of the initial model conditions, parameterization schemes, and/or the inclusion of multiple simulation models, an ensemble of model outputs can be generated to include as much as possible future climate scenarios,” Yang added.
“Existing model ensemble techniques, such as Simple Model Averaging (SMA) and Bayesian Model Averaging (BMA), could reduce single model biases, but these conventional approaches are also associated with many limitations when dealing with nonstationary climate and changing conditions. Alternatively, Machine Learning (ML) tools are more flexible and powerful to do the same job in reducing GCM ensemble biases,” Yang said.
Hence, Dr. Tiantian Yang and his graduate students, Lujun Zhang and Dilip Neupane, from the University of Oklahoma’s School of Civil Engineering and Environmental Science, evaluated the performance of different ML models in reconstructing historical monthly precipitation by averaging multiple GCM simulations over Oklahoma during 1981 to 2014 period and benchmarked the alternative ML approach with the conventional model ensemble techniques
A total of six different model-averaging techniques were employed in this study to combine the 30-member ensemble precipitation simulations from NOAA’s Seamless System for Prediction and EArth System Research (SPEAR) for the reconstruction of monthly precipitation over OK. Employed ML model included the Random Forest (RF), Support Vector Machine (SVM), eXtreme Gradient Boosting (XGBoost), and Classification and Regression Trees (CART). Both spatial and seasonal analyses over OK were conducted with the evaluation statistics of percentage bias, coefficient of determination, and normalized root mean square error.
“We found that the ML-based model ensemble results are superior to the conventional BMA and SMA ensemble techniques as indicated by the employed evaluation statistics. Specifically, in our retrospective studies, the employed MLs bring higher skill scores and lower model biases than the baseline models. Among the employed ML models, the tree-based ML algorithms (RF, CART, and XGB) show slightly better performance than others ML models,” Yang said.
“Strong seasonal and spatial patterns are also observed in terms of the simulation performance over OK. The ML algorithms well represented the seasonal and spatial patterns when compared to the baseline SMA and BMA results. We suspect such performance variation over different space and time in OK are due to a specific precipitation mechanism of mesoscale convective precipitation which surpasses the simulation capability of SPEAR,” Yang said.
This study highlights the great potential of advanced data analytics and ML techniques to further improve the simulation performance of GCM-generated precipitation projections. A research manuscript is being prepared summarizing this seed grant results, and the funded students of this project will present this research at the coming American Geophysical Union (AGU) conference in December 2023 in San Francisco.
____________
This work was initiated with support from the National Science Foundation under Grant No. OIA-1946093 through OK NSF EPSCoR Seed Grant.